Songlin Yang
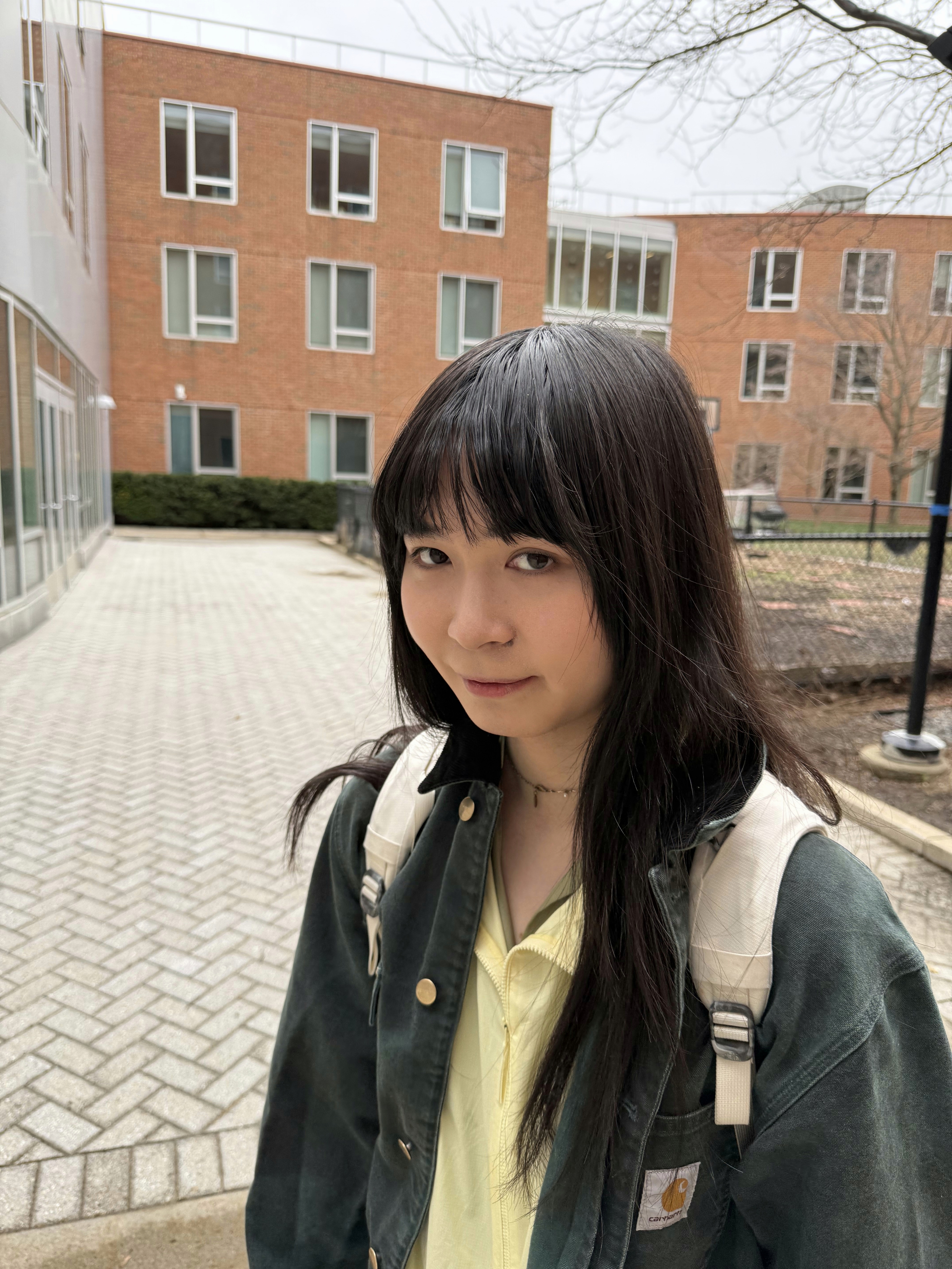
Songlin (松琳) is a second-year PhD student at MIT CSAIL, advised by Prof. Yoon Kim. She earned her bachelor’s degree from SUSTech in 2020 and her master’s degree from ShanghaiTech in 2023, where she was advised by Prof. Kewei Tu.
Her research focuses on the intersection of machine learning systems and large language models, with a particular interest in hardware-aware algorithm design for efficient sequence modeling — especially in linear attention models. For more on her work, see this video and her slides.
She is a strong advocate for open-source research 🐳. Explore the open-source library flash-linear-attention and the fully virtual seminar series Advances in Sequence Modeling from Algorithmic Perspectives — past talks are available here. The best way to reach her is through the FLA Discord Community.
latest posts
Dec 3, 2024 | DeltaNet Explained (Part III) |
---|---|
Dec 3, 2024 | DeltaNet Explained (Part II) |
Dec 3, 2024 | DeltaNet Explained (Part I) |